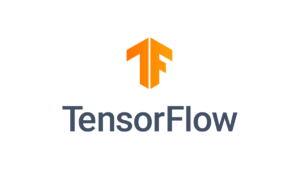
Sentence Transformerの勉強をしている時、テキストの類似度を図る指標としてコサイン類似度が出てきた。
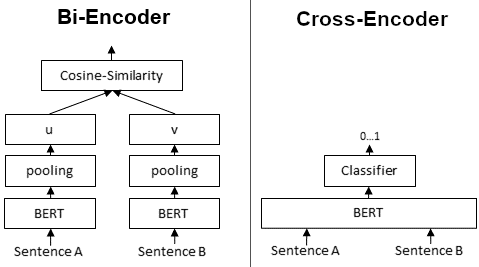
Cross-Encoders — Sentence Transformers documentation
Tensorflowにはコサイン類似度を計算するlossやmetricsはあるが、Layerは存在しない。
tf.keras.losses.cosine_similarity | TensorFlow v2.16.1
Computes the cosine similarity between labels and predictions.
tf.keras.metrics.CosineSimilarity | TensorFlow v2.16.1
Computes the cosine similarity between the labels and predictions.
なので、Bi Encoderを試すために自分で作ってみた。
class CosineSimilarity(tf.keras.layers.Layer):
def __init__(self):
super(CosineSimilarity, self).__init__()
def call(self, x, y):
x = tf.linalg.l2_normalize(x, axis=-1)
y = tf.linalg.l2_normalize(y, axis=-1)
similarity = tf.reduce_sum(x * y, axis=-1)
return similarity
計算があっているかどうか、以下の例で検算してみる。
A = [[0., 1.], [1., 1.], [1., 1.]]
B = [[1., 0.], [1., 1.], [-1., -1.]]
まずはTensorflowのlossから。
loss関数は最小化することを目的として作られているため、符号を反転することに注意。
similarity = -tf.keras.losses.cosine_similarity(A, B, axis=1)
similarity.numpy()
# tf.Tensor([ 0. 0.99999994 -0.99999994], shape=(3,), dtype=float32)
次にscikit-learnで検算。
全ペアの行列で帰ってくるため、対角のみを取得する。
from sklearn.metrics.pairwise import cosine_similarity
similarity = cosine_similarity(A, B)
similarity = np.diag(similarity)
# [ 0. 1. -1.]
最後に自作のレイヤー。
CosineSimilarity()(A, B)
# <tf.Tensor: shape=(3,), dtype=float32, numpy=array([ 0. , 0.99999994, -0.99999994], dtype=float32)>
全ての値が一致したので、自作レイヤーは正しそう。
これで、model内でコサイン類似度を扱うことが出来るようになった。
コメント